Diary of AI CEO [EP.2] : Misconceptions about AI & Data in Business
In the first episode of Diary of an AI CEO, I shared insights on Artificial General Intelligence (AGI). Today, based on my experience engaging with both AI enthusiasts and business leaders seeking to implement AI to enhance organizational processes, I have observed recurring misconceptions regarding AI adoption. Therefore, I have compiled four key topics that I am frequently asked about—both by those around me and by clients. I would like to share these perspectives with readers to provide alternative viewpoints for consideration.
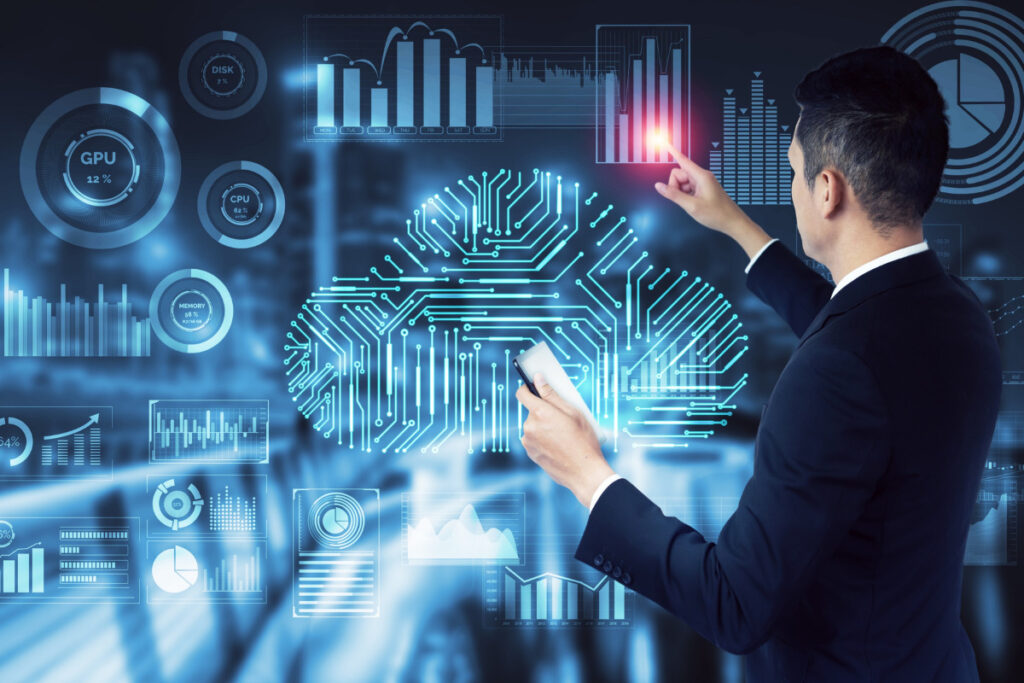
4 Misconceptions about AI & Data in Business
1. Misconceptions about AI & Data in Business
- Data don’t always come where they should. Process understanding is important where, what data and when they become available for AI model, or human-alike, to consume. Often, we collect data in the system which can be fed to AI for training. But in production, in the actual workflow, data has not arrived in time for AI to use then what we trained earlier with the complete final set of data is useless.
- Having a lot of data does not always equate to business gain from that data. Some data might be completely not useable for example unstructured data requires a lot of effort to turn into structured data that can be used for analytics, insight, or for AI to consume. Some has data in quantity but does not have quality, requiring effort to clean up, handle missing data. In the best case when people think they have a lot of data, sometimes these data have no prediction power on what they want to accomplish. Data is then less useful than people take for granted.
2. Mistrust in the AI : always blame AI first even though the problem lies elsewhere.
- Bad input : users scan really bad. But since workers do not see, it didn’t get caught
- The data is simply not present : OCR cannot read these fields → (immediatley conclude)something is wrong with the AI → Finding: it wasn’t on the paper in the first place
- Bug vs. capability limitation → AI cannot perform this task = bug After all, AI is a statistical model. Do not confuse exact-match with best judgment statistically. Sometimes you sacrifice the specific in order to gain the generalization. That is AI.
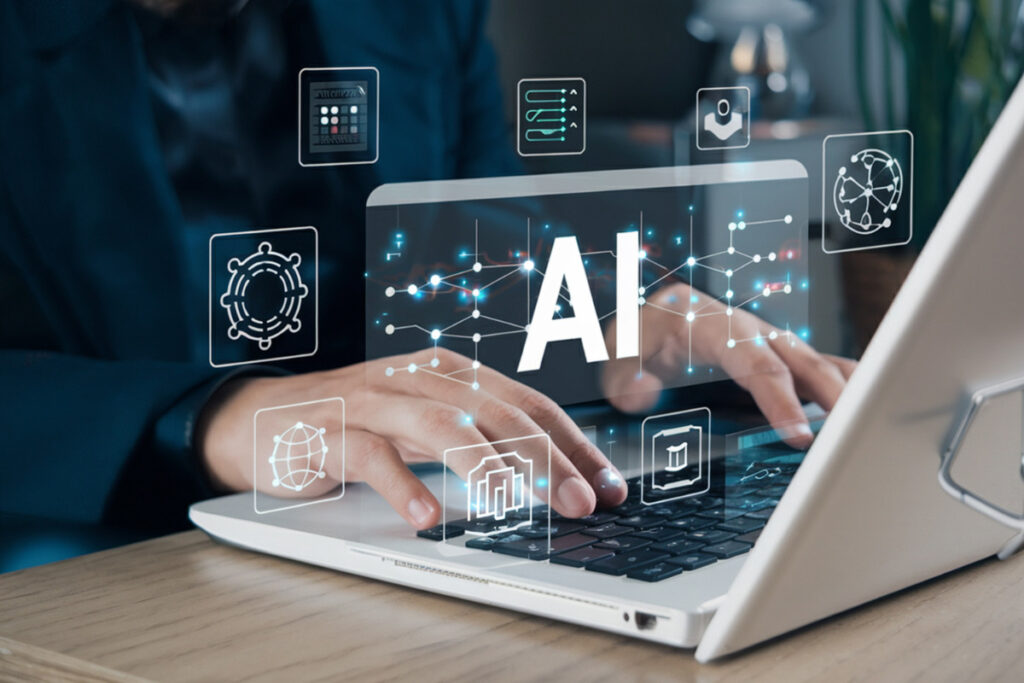
3. AI is just a tool : you have to adapt to it somewhat
- Commandment 1 : If you try to break it, it will.
- Commandment 2 : If you give it unclear input, do not expect it to work well e.g. throw in misinformation or unrelated information to the bot, give input that is really not clear to do OCR
4. Train to 100%
- Remember sometimes if you try to fix something, it will affect other things. Even though overall, by a certain test bench/test set, if selected well, should represent overall improvement. But if you try to specifically get it right on certain thing, you are likely to overfit on the problem and be prepared for it to not generalize well to other problem you may not be aware could happen. Unless you are sure your specific requirement is definite, do not try to overfit an AI to your problem.
- Even with an AI that is, say, 99% accurate, which is a very high number already, many users in order to accept the usage of AI is expecting that AI has to do things all correct simultaneously in order for them to use. For example, the task of AI reading document fields with AI-OCR and all 5 fields have to be correct to the dot and dash. This means the chance of that happening is (99%) to the power of 5 or 95%. Imagine expectation for 10 fields and less than near-perfect accuracy possible of an AI.
Discover unique insights into AI development from the perspective of an AI developer, unlike anything you’ve encountered before, in the upcoming episode of DIARY OF AI CEO. Stay tuned for its release soon. If you have specific topics related to AI development that you would like me to share, feel free to reach out via email at [email protected]. See you in the next episode!
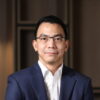
CEO บริษัท ไอเจ็น จำกัด-ผู้เชี่ยวชาญด้าน AI และ Machine learning ทั้งในไทยและต่างประเทศมามากกว่า 10 ปี