Diary of AI CEO [EP.1] : Get to know with “Artificial AGI”
Given the excitement of Generative AI Large-language model (LLM) intelligence and how it can now solve problems, answer questions naturally like humans, words on the street have started to talk about whether we are near AGI or Artificial General Intelligence. AGI can be defined as the AI that is generalized such that it can deduce new knowledge from old ones through reasoning even on the things it has never seen before.
However, despite the incredible smartness we have witnessed of LLMs in recent years, at best these LLMs seem to be able to appear intelligent in so many subject domains because of the way it memorizes patterns from the enormous data it has been trained with. And it does not really use reasoning in creating responses. It is argued here that even if we reached what appears to be AGI through current LLM technology, at best it is only artificial AGI hence the term artificial Artificial General Intelligence in the title (it’s not an editing mistake!)
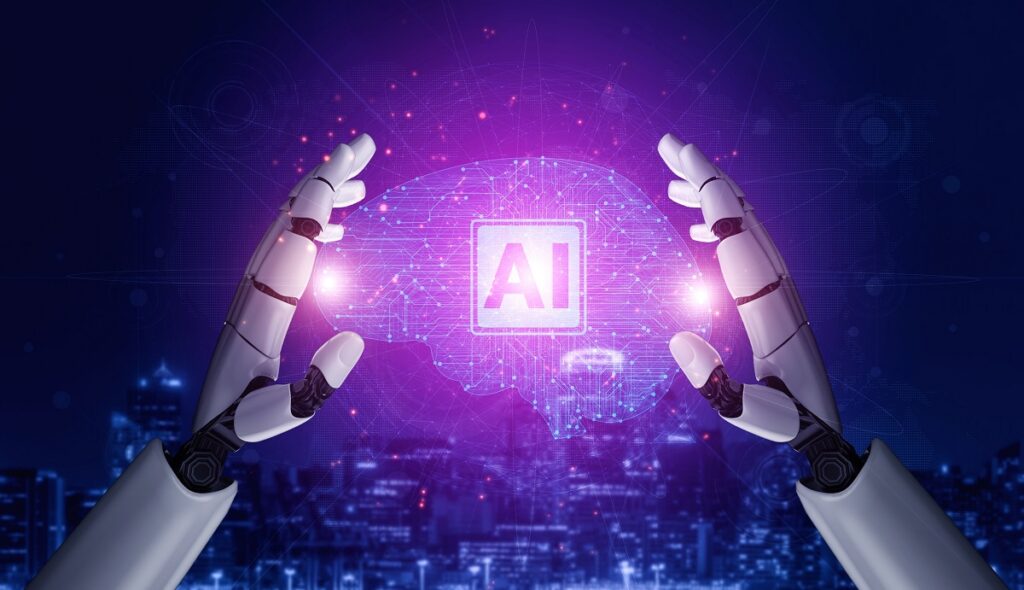
What is Artificial AGI?
Seemingly, these LLM’s appear to be almost like AGI. But in fact, at best it can only be artificial AGI or Artificial Artificial General Intelligence. That is, the true AGI should be able to reason and infer new domain knowledge from existing domain knowledge it was trained with just like humans do when it is still a small child. The way humans learn just by seeing a few examples of new information is still out of reach of AGI for the same computing resource and memory.
It is true that GenAI LLM can seemingly infer correct answers for absolutely new things it has never seen before (zero-shot inference), but the mechanism that it does was simply from seeing many things similar before, not from “reasoning”. As Apple researchers published recently, when changing parameters such as name and number in problem solving type of questions, the performance of the LLM who previously completed the test was better than human. Throwing in unrelated information also confuses the LLM making its performance drop by as much as 17%. In some models, the performance drop can be as high as 65%, showing that it does not really use reasoning in giving out the answer. Humans can handle such variation much better.
Yan LeCunn, one of the father figures of AI, used to say LLM is just a very smart parrot, regurgitating words to respond to query based on patterns and data it has seen before.
Even OpenAI GPT that is claimed to do some reasoning before giving an answer has been given a skeptical look that it is merely an automatic way to do Chain-of-thought query and response. Chain-of-though or CoT is a well-known trick to break down complex problems into smaller tasks and guide the LLM through each task so that it can give better results overall and that GPT-o1 is simply a model that makes these steps automated (remarks: most likely there is more to GPT-o1 than that and this is mere speculation or at least how it appears to work).
If we cannot have AGI, what good will it be?
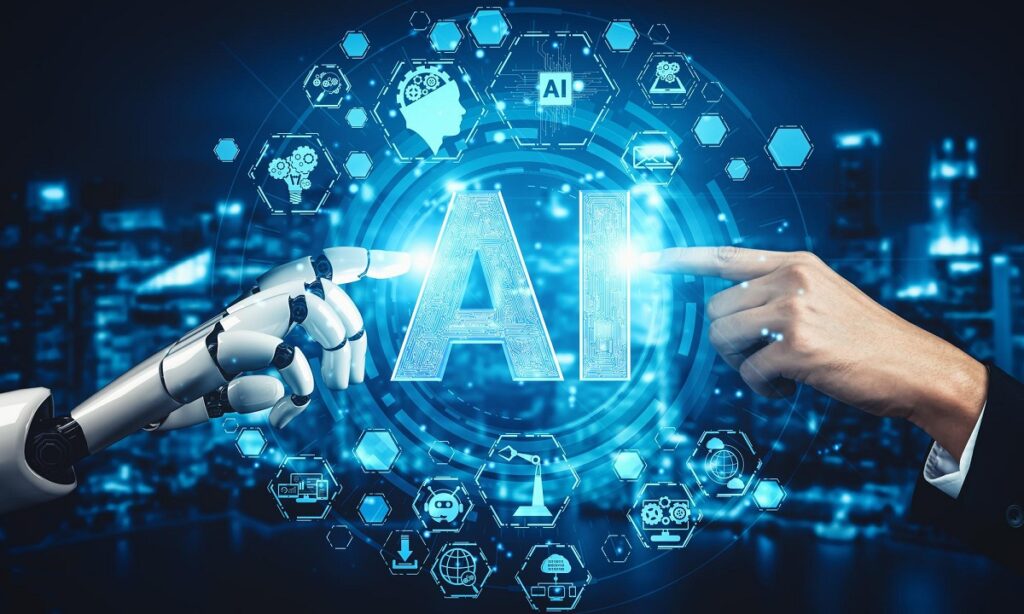
It is worth noting always that when you have a plethora of tools at hand, you choose a tool that is appropriate for the task at hand. If you have a scissor, you wouldn’t try to use it to hammer a nail. Likewise, LLM is already very useful and very powerful. If you use it to summarize reports, write marketing materials, and create well-written email responses, you will get a multi-fold productivity increase. If you use it to solve some math equation, it can probably do ok. If you use it to solve complex problems and try to throw it off, then you wouldn’t get a good result.
Using the tools according to its design will take you a long way. Think of having manuals, or if you don’t like reading manuals, learning by doing is not a bad way to learn about the tool you have. To think of present day AI as an all-knowing do-it-all like AGI might be a glass-half-empty view and you will end up disappointed. But viewing it as simply just a tool, then we will find the way to use this tool appropriately once we understand its nature and its design, and you will end up reaping rewards.
Dismissing real AGI, not so fast …
At this point in time, it is easy to dismiss the notion of AGI as still far-fetched, being only artificial Artificial Intelligence. But given the progress we have made in AI, one should not discount the speed of paradigm shift that we can achieve now. Smart LLM can now be used to augment data, teach or work in tandem with another AI, resulting in exponential increase in the speed of development of everything.
OpenAI-o1 model might be the beginning of such a paradigm shift from data processing AI to reasoning AI. It has been empirically shown somewhat that a reasoning AI that takes time to “think” for 20 seconds more can do the task equivalent to now-old fashion AI (how ironic this word sounds is hard to describe. It’s like an oxy-moron.) can do with 100,000 times more data in training and 100,000 times larger model [ref]. This made such a model in this new paradigm more suited to complex problem solving tasks as it becomes closer to how human thinking and reasoning works.
We might be on the cusp of AGI if the promising evidence of how o1 model can beat the old-fashion gpt4 model and the speed of development pan out as projected but this is yet to be seen. As they said, shoot for AGI, the worst you could end up with is artificial AGI.
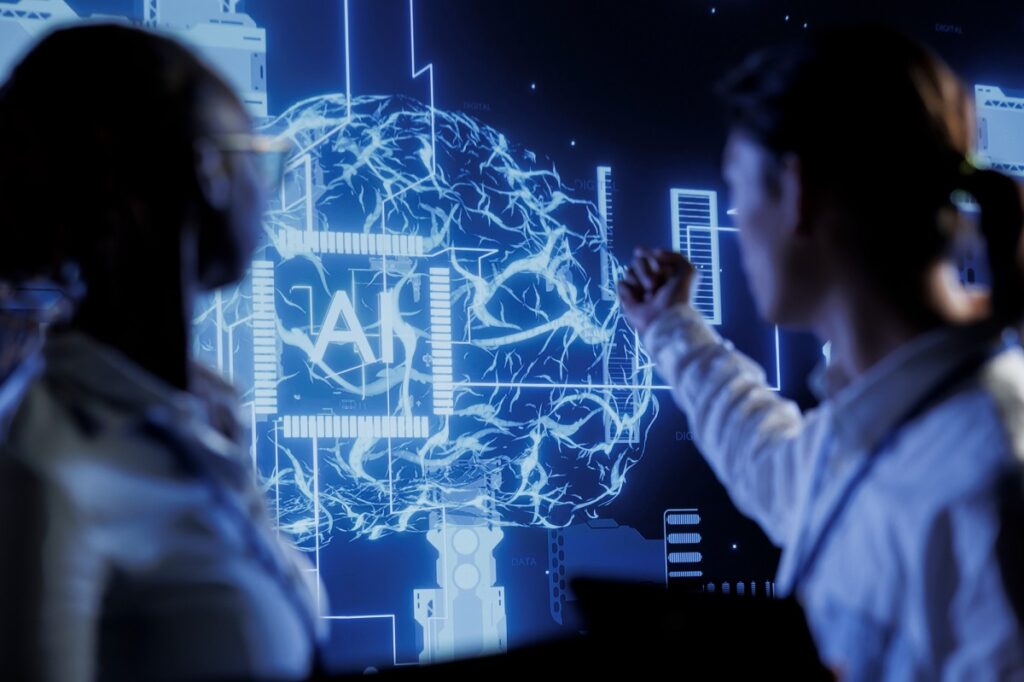
Bonus Materials
Here are a few notable fine prints that might appear on such manuals of present day AI:
- LLM can still make mistakes. Just like humans do. Mistakes come in many forms: incorrect answers (think of incompetent human being who sometimes is so sure of his answer), making things up or hallucinations (some people also do that, mixing things up that he inserts non-existing information into the content. Being a believable guy, speaking with a confident loud voice, people would believe he knows his stuff!) or be rude and socially inappropriate at times.
- Guiding them through a thought process, Chain-of-though or CoT as it is called, may help you get a better final result.
- Suddenly changing subjects can confuse AI as much as you can confuse your human conversant. Try not to mix it up too much. That’s why there is a “New Chat” for every commercial Generative AI.
The list can go on and on but this already assumes most people already know the basics of how to “prompt” or even a little more advanced prompt engineering lessons given all over the Internet.
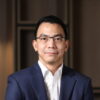
CEO บริษัท ไอเจ็น จำกัด-ผู้เชี่ยวชาญด้าน AI และ Machine learning ทั้งในไทยและต่างประเทศมามากกว่า 10 ปี